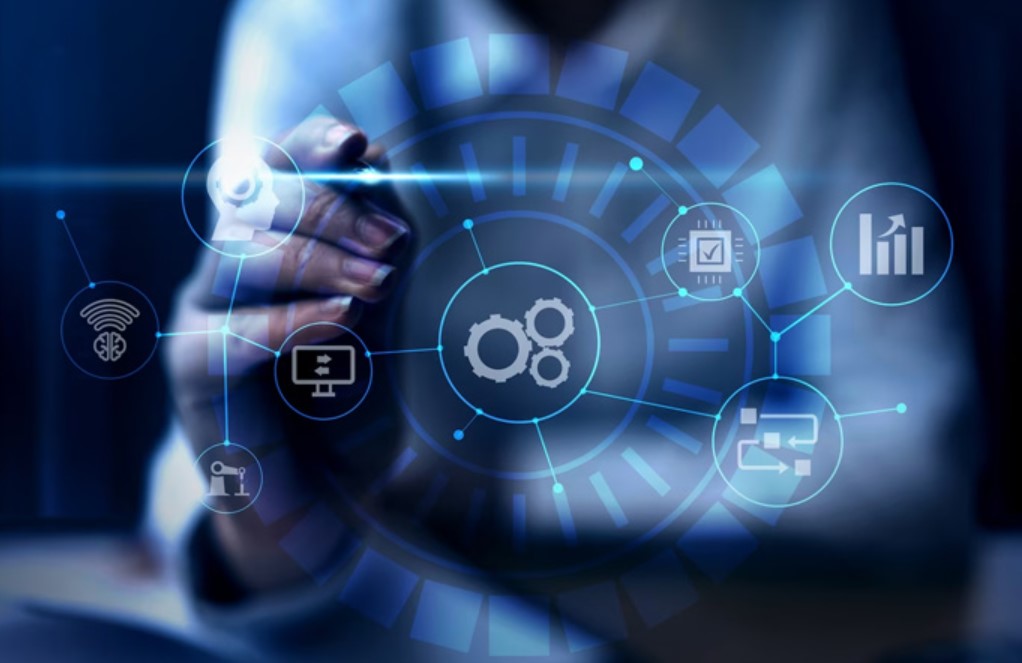
Implementing A/B Testing and Controlled Experiments for Data-Driven Decision-Making
Introduction
In the world of data science, A/B testing and controlled experiments are powerful tools for data-driven decision-making. These methodologies enable organisations to scientifically test different strategies and interventions, allowing them to determine the most effective approach for improving key performance metrics. Whether you are testing website designs, marketing strategies, or product features, A/B testing can help optimise your decision-making process. If you are interested in diving deeper into such methodologies, a Data Analyst Course can provide you with the skills and knowledge to execute such experiments.
Understanding A/B Testing
A/B testing is a closely controlled experiment where two versions (A and B) of a variable are compared to identify the one that performs better in predefined metrics. It is commonly used in digital marketing, web design, product development, and UX optimisation, among other areas. In an A/B test, the population is divided into two groups, each exposed to a different variant. The outcomes are then analysed to identify statistically significant differences between the two. To master these techniques, professionals should commit themselves to a systematic learning program, such as enrolling in a well-structured data course such as a Data Analytics Course in Mumbai and such reputed learning hubs.
Key Concepts in A/B Testing:
- Control Group (A): The original version or baseline represents the existing conditions.
- Treatment Group (B): This is the new version or intervention that is being tested.
- Randomisation: Participants are randomly assigned to either group to avoid bias and ensure the results are reliable.
- Metrics: Key performance indicators (KPIs), such as conversion rate, click-through rate, or customer engagement, that will help measure the success of the test.
Designing A/B Tests
Properly designing an A/B test is critical for obtaining accurate and meaningful results. Below are the steps involved in designing a robust A/B test:
Define the Hypothesis
Before conducting the test, it is essential to clearly define the hypothesis you want to test. A hypothesis is a statement that predicts the outcome of the experiment. For example, if you are testing a new button design for your website, the hypothesis could be: “Changing the colour of the CTA (Call to Action) button from blue to green will increase the click-through rate by 5%.”
Set Clear Metrics for Success
Choose the right KPIs that align with your business objectives. In our example, the conversion rate or click-through rate (CTR) would be the relevant metric. Measuring both primary and secondary outcomes is important, as changes in user behaviour might affect multiple metrics. A Data Analyst Course typically covers a solid understanding of metrics, ensuring that you apply the right analysis techniques.
Randomisation and Segmentation
Randomly assign users to either the control or treatment group. This helps eliminate selection bias and ensures that the experiment results are statistically valid. In some cases, user segmentation may be appropriate to ensure that the test accounts for different types of users, such as new visitors versus returning customers.
Control Confounding Variables
Controlling for confounding variables—external factors that may influence the results of the experiment, such as seasonal trends or sudden market shifts—is essential to ensure that differences observed in outcomes are caused by the changes made in the test.
Statistical Significance and Sample Size
A core aspect of A/B testing is ensuring that the results are statistically significant. This means that any differences observed between the control and treatment groups are unlikely to have occurred by chance.
Sample Size Calculation
You can use statistical power analysis to determine the minimum sample size needed for a valid experiment. This ensures that the test has enough power to detect meaningful differences between the groups. Factors such as effect size, significance level (usually 0.05), and desired power (typically 80%) must be considered when calculating the sample size.
Statistical Tests
Once the data is collected, statistical tests such as t-tests or chi-square tests compare the control and treatment groups. A p-value is calculated to determine if the difference between the groups is statistically significant. If the p-value is less than the chosen significance level (usually 0.05), the null hypothesis (no difference between the groups) is rejected.
Best Practices for A/B Testing
To ensure your A/B tests yield reliable and actionable results, it is important to follow a few best practices. A career-oriented data course such as a professional-level Data Analytics Course in Mumbai will include several assignments that will acquaint learners with such best practice guidelines.
Test One Variable at a Time
One of the golden rules of A/B testing is to change only one variable between the two groups. If you test multiple changes at once, it becomes difficult to attribute any differences in outcomes to a specific change.
Ensure Sufficient Test Duration
Run the test for an adequate amount of time to gather enough data. Testing for too short a period may lead to inconclusive results due to sample size limitations or external factors like seasonal traffic fluctuations. On the other hand, excessively long tests might expose the experiment to biases introduced by changes in external conditions over time.
Monitor for Biases
While conducting the test, it is important to monitor for biases. For example, if users are not randomly assigned or if external events influence one group more than the other, the results may not be valid. Regular checks need to be conducted to ensure that the randomisation and sample selection process are unbiased.
Post-Test Analysis
Once the test is complete, analyse the results carefully. If the test confirms the hypothesis, it may be appropriate to implement the change across all users. However, if the results are inconclusive or do not support the hypothesis, it may be necessary to rethink the strategy or conduct further tests.
Challenges in A/B Testing
Despite its effectiveness, A/B testing comes with several challenges:
Test Duration and Seasonal Variability
In some industries, seasonal changes or marketing campaigns can limit the appropriate time to run tests. For example, if you test a website redesign during the holiday season, the results may not be applicable for the rest of the year. It is important to account for such external factors to ensure the validity of the test.
Traffic Limitations
Running A/B tests may not yield enough data for reliable results in cases where traffic is limited. Low-traffic websites or small user bases may require larger sample sizes or more time to run the test effectively.
Confounding Factors
As mentioned earlier, external variables such as competitor actions, market trends, or even internal events like site outages can interfere with test results. Ensuring that the experiment is conducted under controlled and stable conditions is essential for obtaining accurate data.
Advanced Approaches: Multivariate Testing and Sequential Testing
While A/B testing is effective, more advanced techniques, such as multivariate and sequential testing, can offer more nuanced insights in certain situations.
Multivariate Testing
In multivariate testing, instead of comparing just two versions (A vs. B), multiple variables or combinations of variables are tested simultaneously. This allows you to determine which combination of factors results in the best performance. For example, testing different combinations of images, button placements, and text on a landing page.
Sequential Testing
Sequential testing analyses results as they come in rather than waiting for a fixed sample size. This method allows for faster decision-making and can help avoid wasting time and resources on tests that are unlikely to produce significant results.
Implementing the Results
After completing the A/B test, the results should guide decision-making. If the treatment group (B) outperforms the control group (A), consider implementing the winning variation across your user base. If the results are inconclusive, refining the hypothesis and iterating on the test design is important. Continuous experimentation is key to optimising business processes and improving customer satisfaction. Learning to properly implement these results is an essential skill taught in any inclusive data course; for example; a Data Analytics Course in Mumbai and such urban learning hubs where these courses are tailored for professionals.
Conclusion
A/B testing and controlled experiments are essential components of data-driven decision-making. By carefully designing, running, and analysing A/B tests, businesses can derive insights that lead to better customer experiences, optimised marketing strategies, and improved product offerings. The critical factors for successful A/B testing include clear hypothesis formulation, proper randomisation, valid sample sizes, and statistical analysis. With these methodologies in place, organisations can make more informed decisions and achieve measurable improvements across various domains. For anyone looking to refine their understanding of these methodologies and more, a Data Analyst Course offers invaluable insights into the world of data experimentation.
Business name: ExcelR- Data Science, Data Analytics, Business Analytics Course Training Mumbai
Address: 304, 3rd Floor, Pratibha Building. Three Petrol pump, Lal Bahadur Shastri Rd, opposite Manas Tower, Pakhdi, Thane West, Thane, Maharashtra 400602
Phone: 09108238354
Email: [email protected]