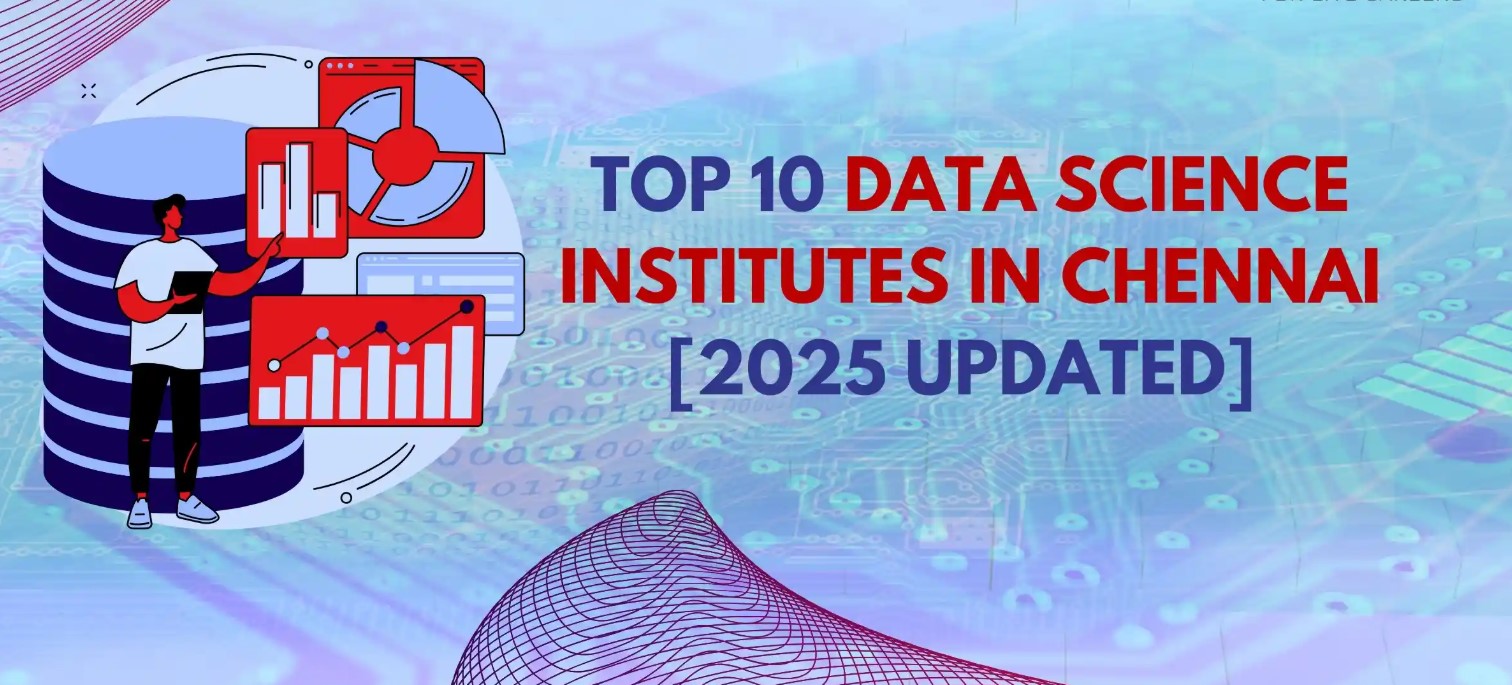
Implementing Active Learning for Efficient Data Labeling
Data labeling is a critical component in the development of machine learning models. It involves annotating data to provide the ground truth needed for training algorithms. However, manual data labeling is often labor-intensive, time-consuming, and expensive. Active learning, a subfield of machine learning, addresses these challenges by selecting the most informative data points for labeling, thereby optimising the labeling process. Understanding active learning is essential for students taking a Data Science Course in Chennai to improve data efficiency and model performance.
What is Active Learning?
Active learning is a continual process in which an ML model actively selects the most instructive samples from an unlabeled dataset for annotation. This approach contrasts with passive learning, where the model is trained on an arbitrarily selected subset of data. In a Data Science Course in Chennai, students learn that active learning aims to achieve higher accuracy with fewer labeled examples, making it a cost-effective strategy for data labeling.
The Importance of Active Learning
The primary advantage of active learning is its ability to reduce the amount of labeled data required to train a high-performing model. This is particularly beneficial when labeling is expensive or labor-intensive, such as medical imaging or natural language processing. By focusing on the most uncertain or diverse samples, active learning ensures that each labeled example contributes maximally to the model’s learning process. A Data Science Course in Chennai emphasises these benefits, highlighting how active learning can lead to high cost and time savings in real-world applications.
Active Learning Strategies
Several strategies are used in active learning to select the most informative data points. Standard methods include uncertainty sampling, query-by-committee, and diversity sampling.
- Uncertainty Sampling: This method involves selecting samples for which the model is least confident in its predictions. For instance, in a Data Science Course, students might learn to use techniques like entropy, margin sampling, or least confident sampling to identify these uncertain samples.
- Query-by-Committee: In this approach, multiple models (a committee) are trained on the same data and the samples on which the models disagree the most are selected for labeling. This method ensures that the selected samples are the most contentious and informative.
- Diversity Sampling: This strategy focuses on selecting a diverse set of samples to ensure that the labeled dataset covers a wide range of the input space. Techniques such as clustering or core-set selection are often used to achieve this diversity.
Understanding these strategies is a critical component of a Data Science Course, where students are trained to apply the correct method based on the specific requirements of their projects.
Implementing Active Learning in Practice
Implementing active learning involves several steps, including initialising the model, selecting samples, labeling, and updating the model. Here is a practical guide to implementing active learning:
- Initialise the Model: Start with a small, labeled dataset to train an initial model. In a Data Science Course in Chennai, students learn the importance of this step in providing a foundation for active learning.
- Select Samples: Use an active learning strategy to choose the most informative samples from the unlabeled dataset. This step is crucial for optimising the labeling process.
- Label the Selected Samples: These samples are then labeled to human annotators. The efficiency gained in this step is a critical focus in a Data Science Course in Chennai, where students explore methods to streamline the annotation process.
- Update the Model: Retrain the model with the newly labeled data and repeat the process. Each iteration improves the model’s performance while minimising the labeled data required.
Challenges and Solutions in Active Learning
While active learning offers many benefits, it also presents several challenges. One significant challenge is the initial selection of the labeled dataset, as a poor initial set can lead to suboptimal model performance. To address this, students in a Data Science course are taught to use techniques like random sampling or domain-specific heuristics to create a robust initial dataset.
Another challenge is the potential for labeling bias, where the selected samples may only represent some of the datasets. Diversity sampling and hybrid approaches combining multiple strategies are often employed to mitigate this. These solutions are covered extensively in a Data Science Course, preparing students to handle real-world data labeling challenges effectively.
Applications of Active Learning
Active learning is widely used across various domains. In healthcare, it aids in annotating medical images and clinical texts, improving diagnostic models. In the automotive industry, active learning helps label sensor data for autonomous vehicles. For students in a Data Science Course, these applications demonstrate the practical importance of active learning in developing efficient and accurate machine learning models.
Conclusion: Active learning is a powerful tool for efficient data labeling, reducing the costs and time associated with manual annotation. Focusing on the most informative samples ensures that machine learning models achieve high performance with fewer labeled examples. For those pursuing a Data Science Course in Chennai, mastering active learning techniques is crucial for advancing in data science and artificial intelligence. As the demand for efficient data labeling grows, the ability to implement active learning will become an increasingly valuable skill in the data scientist’s toolkit.
BUSINESS DETAILS:
NAME: ExcelR- Data Science, Data Analyst, Business Analyst Course Training Chennai
ADDRESS: 857, Poonamallee High Rd, Kilpauk, Chennai, Tamil Nadu 600010
Phone: 8591364838
Email- [email protected]
WORKING HOURS: MON-SAT [10AM-7PM]